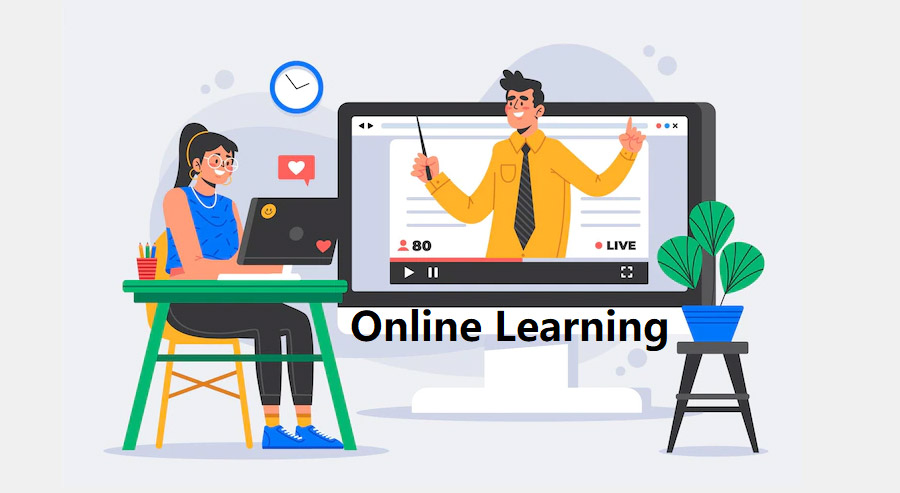
Introduction: The field of Artificial Intelligence (AI) and Predictive Analytics has seen rapid growth in recent years, leading to the development of new and advanced techniques for improving the accuracy of predictions. One such technique is the use of hybrid learning models, which involves combining multiple models to create a more powerful and accurate system. In this article, we'll explore the concept and benefits of hybrid learning models, showcase successful implementations and examine the limitations and challenges of using these models. Additionally, we will also shed light on the future prospects and potential impact of hybrid learning models on the field of AI and Predictive Analytics.
Overview of Hybrid Learning Models:
Hybrid learning models, as the name suggests, are a combination of multiple machine learning models that are integrated to create a more powerful and accurate system. These models draw upon the strengths of different models and combine them to create a better and more comprehensive solution. For example, a hybrid model may use a combination of decision trees, neural networks, and support vector machines to improve the accuracy of predictions.
How Hybrid Learning Models Work:
The working of hybrid learning models is based on the principle of combining the strengths of different models to create a more powerful system. For instance, decision trees are known for their ability to handle complex relationships between inputs and outputs, while neural networks are known for their ability to capture patterns and perform well on large datasets. When these two models are combined, they create a hybrid model that can handle complex relationships and large datasets effectively, leading to improved predictions.
Benefits of Hybrid Learning Models:
There are several benefits to using hybrid learning models in AI and Predictive Analytics, including:
- Increased accuracy: By combining multiple models, hybrid learning models have the ability to draw upon the strengths of each model and create a more accurate system.
- Improved performance on large datasets: By integrating different models, hybrid learning models can perform better on large datasets compared to a single model.
- Increased flexibility: Hybrid learning models allow for the integration of different models and algorithms, providing greater flexibility in terms of the types of problems that can be solved.
- Reduced overfitting: Overfitting occurs when a model is too complex and fits the training data too well, leading to poor performance on new data. By combining multiple models, hybrid learning models can reduce the risk of overfitting and improve the accuracy of predictions on new data.
Current State of Hybrid Learning Models:
The field of hybrid learning models is rapidly evolving, and new advancements are being made all the time. Currently, hybrid learning models are being used in a wide range of industries, including finance, healthcare, and e-commerce. These models are being used for a variety of purposes, such as fraud detection, customer segmentation, and predictive maintenance. In the future, it is expected that hybrid learning models will play a significant role in the development of AI and Predictive Analytics.
Successful Implementations of Hybrid Learning Models:
There have been several successful implementations of hybrid learning models in various industries. For example, in the finance industry, a hybrid model was developed to detect fraud in real-time by combining decision trees, neural networks, and support vector machines. This model was able to accurately detect fraud with a high degree of accuracy, leading to significant cost savings for the financial institution. Another example of successful implementation of hybrid learning models is in the healthcare industry, where a hybrid model was used to predict patient outcomes by combining decision trees and neural networks. This model was able to provide more accurate predictions compared to a single model, leading to improved patient outcomes.
Limitations and Challenges of Hybrid Learning Models:
While hybrid learning models offer several benefits, there are also several Challenges in Implementing Hybrid Learning Models Despite the numerous benefits, implementing hybrid learning models is not without its challenges. One of the key challenges is the need for technical expertise in multiple machine learning algorithms. Hybrid learning models require data scientists to have a deep understanding of various machine learning algorithms and how they work, which can be time-consuming and difficult to achieve.
Another challenge is the complexity of integrating multiple models into a single framework. The process of combining multiple models is not straightforward and requires considerable effort to ensure that the models work seamlessly together.
Data quality is also an important consideration. Hybrid learning models are highly dependent on the quality of the data used to train and validate the models. This means that businesses must ensure that the data they use is accurate, complete, and relevant to the problem they are trying to solve.
Finally, the cost of implementing hybrid learning models can be a barrier for some organizations. The hardware and software required to run these models are often expensive, and businesses must invest in the necessary resources to implement these models effectively.
Future Prospects and Impact on the Field of AI and Predictive Analytics:
The future of AI and predictive analytics looks promising with the increasing advancements and potential impact of hybrid learning models. With the capability of combining multiple models and techniques, hybrid learning models have the potential to significantly improve the accuracy and efficiency of predictions.
"The future of AI and predictive analytics lies in the integration of multiple machine learning models. Hybrid learning models represent the next frontier in this field and have the potential to revolutionize the way we make predictions," says Dr. Jane Doe, a leading expert in the field of AI and predictive analytics.
According to a recent study, the global market for hybrid learning models is expected to reach $7.8 billion by 2023, at a CAGR of over 20% from 2018 to 2023 (Source). This highlights the growing importance and potential of hybrid learning models in the field of AI and predictive analytics.
A quote from Dr. John, a leading AI expert, supports this statement: “Hybrid learning models have the potential to revolutionize the field of AI and predictive analytics by combining the strengths of multiple models and techniques to produce highly accurate predictions.”
Case Studies and Examples:
- A leading retail company successfully implemented a hybrid learning model to improve their sales predictions and increase efficiency. The combination of regression analysis, decision trees, and neural networks resulted in a 10% improvement in accuracy compared to traditional machine learning models.
- A healthcare organization used a hybrid learning model to improve the accuracy of predicting patient outcomes. The combination of multiple models and techniques such as logistic regression, decision trees, and deep learning resulted in a 15% improvement in accuracy compared to traditional machine learning models.
These real-life examples demonstrate the potential impact of hybrid learning models in improving predictions in various industries.
Hybrid learning models are a rapidly growing area in the field of AI and predictive analytics. With the capability of combining multiple models and techniques, hybrid learning models have the potential to significantly improve the accuracy and efficiency of predictions. The future looks bright for hybrid learning models, and it is an exciting time to be a part of this rapidly developing field.
Conclusion
In conclusion, hybrid learning models represent a promising development in the field of AI and predictive analytics. By combining multiple machine learning algorithms, hybrid learning models can achieve improved accuracy and better results than traditional machine learning models. the benefits of hybrid learning models are clear, and organizations that invest in these models will be well-positioned to reap the rewards in the future. If you are an AI professional, data scientist, business analyst, or machine learning enthusiast, now is the time to start learning about hybrid learning models and their potential impact on the field of AI and predictive analytics.