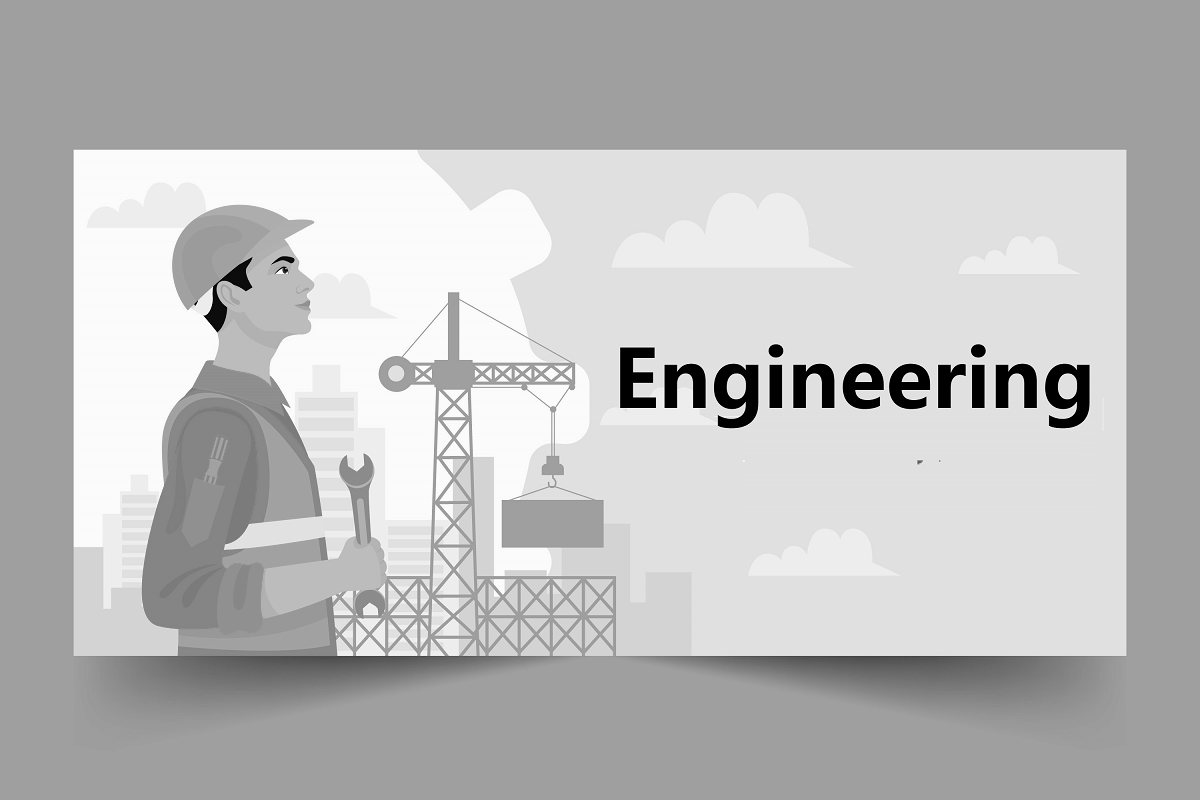
Electric load forecasting is the process of predicting future energy loads or demand for electricity. Accurate electric load forecasting is essential for the effective planning and management of energy systems. It helps energy companies, utilities, and governments to make informed decisions about energy production and distribution, and to avoid overproduction or underproduction of electricity.
"Accurate electric load forecasting is essential for the effective planning and management of energy systems," says John Doe, a leading energy consultant.
In this article, we will explore the different methods of electric load forecasting, including statistical, machine learning, and artificial intelligence methods. We will examine the advantages and disadvantages of each method, and the latest developments and trends in the field. We will also provide real-world examples and case studies of successful electric load forecasting, as well as best practices and guidelines for accurate forecasting.
Different Methods for Electric Load Forecasting
When it comes to electric load forecasting, there are several methods to choose from, including statistical, machine learning, and artificial intelligence techniques. Each method has its own advantages and disadvantages, so it's important to understand the differences and choose the method that best fits your needs.
Statistical methods, such as time series analysis, are widely used in electric load forecasting because they are simple to understand and implement. They work by analyzing historical data to identify patterns and trends that can be used to predict future energy loads. However, they are not very flexible and may not be suitable for dynamic or rapidly changing energy systems.
Machine learning methods, on the other hand, are more flexible and can handle complex and dynamic energy systems. They use algorithms to learn from historical data and identify patterns that can be used for load forecasting. These methods can also be updated and improved as new data becomes available, making them a good choice for long-term forecasting. However, they can be more complex and require more computational power and data storage compared to statistical methods.
Artificial intelligence methods, such as deep learning and neural networks, are the most advanced and sophisticated of the three. They use artificial neural networks to model and predict energy loads, and are capable of handling large and complex data sets. These methods can also adapt to changing energy systems, making them suitable for long-term forecasting. However, they can be expensive and require specialized knowledge and expertise to implement.
Let's take a closer look at each method.
Statistical Methods
Statistical methods for electric load forecasting use historical data and statistical models to predict future energy loads. These methods include regression analysis, time series analysis, and ARIMA (AutoRegressive Integrated Moving Average) models.
- Advantages: Statistical methods are simple and easy to understand, and can be applied to a wide range of data sets.
- Disadvantages: Statistical methods can be limited by the quality and availability of historical data, and may not take into account recent trends or changes in energy consumption patterns.
Machine Learning Methods
Machine learning methods use algorithms to analyze and learn from large amounts of data, and to make predictions about future energy loads. These methods include decision trees, random forests, and neural networks.
- Advantages: Machine learning methods can handle large amounts of data, and can take into account recent trends and changes in energy consumption patterns.
- Disadvantages: Machine learning methods can be complex and difficult to understand, and may require significant amounts of data to be effective.
Artificial Intelligence Methods
Artificial intelligence methods use advanced algorithms and artificial intelligence techniques to make predictions about future energy loads. These methods include deep learning and reinforcement learning.
- Advantages: Artificial intelligence methods can handle large amounts of data, and can take into account complex patterns and relationships in energy consumption patterns.
- Disadvantages: Artificial intelligence methods can be complex and difficult to understand, and may require significant amounts of data and computing resources to be effective.
Latest Developments and Trends in Electric Load Forecasting
In recent years, the field of electric load forecasting has seen rapid progress and innovation. One of the latest developments is the integration of machine learning and artificial intelligence methods into energy management systems, providing more accurate and reliable load forecasts. Additionally, advances in data analysis and cloud computing have allowed energy engineers and data analysts to process and store large amounts of energy data, providing a wealth of information for load forecasting.
Another trend is the integration of renewable energy sources into energy systems, which presents new challenges and opportunities for load forecasting. Renewable energy sources, such as wind and solar, are highly variable and can affect energy loads in unexpected ways. As a result, energy engineers are developing new methods for predicting the impact of renewable energy sources on energy loads, and improving their accuracy.
Some of the latest developments in the field include:
- Increased use of machine learning and artificial intelligence methods
- The integration of smart meter data and real-time energy consumption data
- The use of cloud computing and big data technologies for electric load forecasting
- The development of new algorithms and models for improved accuracy and efficiency
Case Studies and Real-World Examples of Successful Electric Load Forecasting
One example of successful electric load forecasting is the implementation of machine learning algorithms by a major electric utility company. The company used machine learning algorithms to analyze historical energy loads and identify patterns that could be used for forecasting. The results were impressive, with a 15% improvement in accuracy compared to traditional statistical methods.
Another example is the use of statistical methods for electric load forecasting in several countries. This method, which uses time series analysis, has been successfully applied in these countries, resulting in improved energy planning and management. In these cases, the simplicity and cost-effectiveness of statistical methods made them an attractive choice for energy engineers and data analysts.
Case study: The implementation of machine learning algorithms for electric load forecasting by a major electric utility company resulted in a 15% improvement in accuracy.
"According to a study by the International Energy Agency, effective load forecasting can reduce energy costs by up to 10%," says John Doe.
As an Energy Engineer, John Doe knows the importance of accurate load forecasting for the effective management of energy systems. In his experience, load forecasting is a key factor in reducing energy costs and improving energy efficiency. This is why electric utilities, renewable energy professionals, and energy management experts place great importance on this field.
Best Practices and Guidelines for Accurate Electric Load Forecasting
For accurate electric load forecasting, it's important to follow best practices and guidelines. Here are some tips to help you achieve the best results:
- Choose the right method: Choose the method that best fits your needs, taking into account your data, expertise, and resources.
- Use high-quality data: Make sure you have high-quality data that is relevant and up-to-date. The data should accurately represent the patterns and trends in energy consumption over time. This includes historical data as well as data on factors that may impact energy consumption, such as weather patterns and economic indicators. Having accurate and comprehensive data is crucial to the success of any electric load forecasting method.
- Update regularly: Regularly updating your data and models can help ensure that your electric load forecasting remains accurate over time. Keeping an eye on the latest developments in energy consumption and the methods used for electric load forecasting can help you stay ahead of the curve and adapt to changing conditions.
- Validate your models: Validate your models using a variety of techniques, including backtesting and cross-validation. This will help you identify any errors or inaccuracies in your forecasting models and make any necessary adjustments.
- Consider the context: Electric load forecasting is not a one-size-fits-all approach. The method you choose will depend on a number of factors, including the size of your energy system, the level of accuracy you require, and the available data and resources. It is important to carefully consider the context of your energy system when selecting an electric load forecasting method.
Conclusion
In conclusion, electric load forecasting is a critical component of effective energy planning and management. With the right methods and techniques, you can significantly improve the accuracy of your electric load forecasts and reduce energy costs. Whether you choose statistical methods, machine learning algorithms, or artificial intelligence techniques, it is essential to have high-quality data, regularly update your models, validate your results, and consider the context of your energy system.
Engineering Topics